Table of Contents
Introduction
Investors embrace AI and ML to gain a competitive edge in financial markets. These technologies revolutionize growth stock analysis, uncovering hidden patterns and predicting market trends with unprecedented accuracy. As Africa experiences rapid economic growth, the demand for effective investment strategies rises. Growth stocks offer high revenue and earnings growth potential, but navigating their complexities can be daunting. This article explores the intersection of AI, ML, and growth stock investing in Africa, providing a comprehensive guide to leveraging these technologies for investment success.
Introduction to Artificial Intelligence and Machine Learning to Analyze Growth Stock
AI and ML have revolutionized financial markets, enabling investors to process vast data and accurately identify growth opportunities. These technologies analyze historical stock data, financial statements, and even alternative data sources such as social media sentiment to predict the future performance of stocks. In third-world countries, where market information may be less transparent, AI and ML can be particularly beneficial in uncovering growth potential that might otherwise be overlooked.
Overview of Growth Stock Investing
Growth stock investing involves purchasing shares in companies anticipated to grow faster than the market average. These companies usually reinvest their earnings to fuel expansion, focusing on increasing revenue and market share rather than distributing profits as dividends. While growth stocks can offer substantial returns, they also have heightened risks, particularly in unpredictable markets like Africa. Investors may face significant volatility, as the value of these stocks can fluctuate more dramatically due to market conditions and company performance. Careful research and a long-term investment horizon are crucial when considering growth stocks.
The Rise of AI and ML in Financial Markets
The rise of AI and ML in financial markets has revolutionized the investment landscape, democratizing access to sophisticated tools previously available only to large institutions. These technologies have empowered small-scale investors, including those in African countries, to make more informed and strategic investment decisions. AI and ML algorithms quickly analyze vast amounts of data, uncovering patterns and insights that traditional analysis methods might overlook. This allows investors to identify promising growth stocks more accurately, even in complex and volatile markets. As a result, AI and ML are transforming how investments are approached, offering new opportunities for enhanced returns.
How AI and ML Can Enhance the Analysis of Growth Stocks
AI and ML algorithms can analyze complex datasets, uncovering patterns and trends that human analysts might miss. These technologies can predict stock performance, assess market sentiment, and optimize investment portfolios, offering a significant advantage in growth stock analysis.
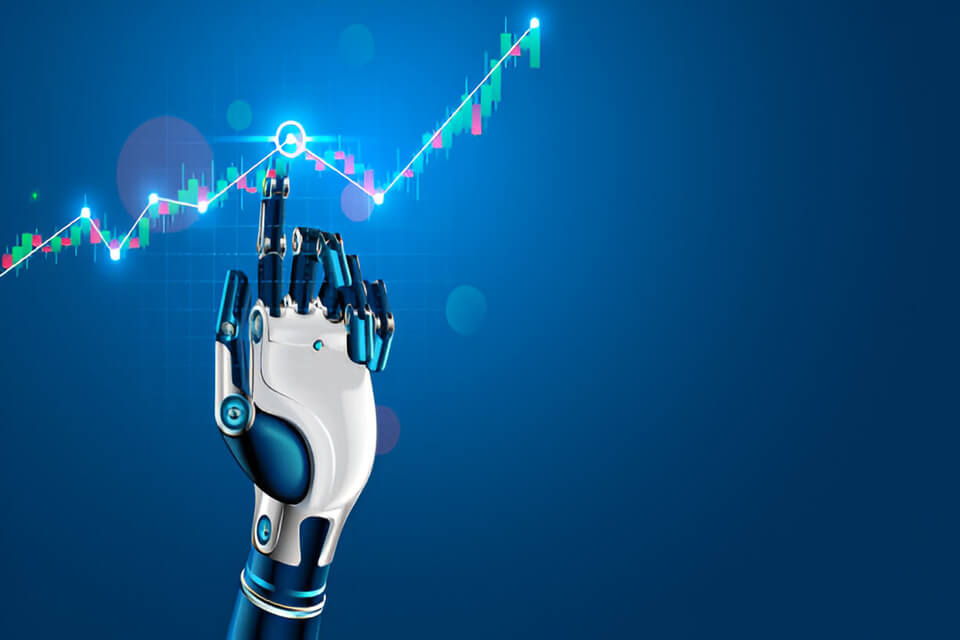
Understanding Growth Stocks
Growth stocks represent companies with the potential for significant revenue and earnings growth. These stocks are often found in innovative sectors such as technology and biotechnology, where companies reinvest profits to fuel further expansion.
Definition and Characteristics of Growth Stocks
- High Revenue and Earnings Growth Potential
Growth stocks are anticipated to outpace the broader market by delivering superior revenue and earnings growth over time. These stocks typically represent companies with innovative products, strong market demand, and robust business models. As a result, they attract investors seeking substantial returns, driven by the potential for accelerated financial performance.
- Reinvestment of Profits for Expansion
Companies often reinvest profits into the business to fuel further expansion rather than distributing dividends to shareholders. This reinvestment strategy is focused on enhancing product development, entering new markets, improving infrastructure, and driving innovation. Companies aim to increase long-term shareholder value by prioritising growth over immediate payouts.
- Technology, Biotech, and Innovative Sectors
The technology, biotech, and innovative sectors are renowned for their rapid pace of innovation and significant growth potential. Companies in these industries frequently develop groundbreaking products and services, capturing market share quickly. Their focus on research and development drives continuous advancements, attracting investors who seek high returns from cutting-edge, transformative business ventures.
Risks and Rewards of Investing in Growth Stocks
- High Volatility and Potential for Significant Returns
Growth stocks, known for their potential to deliver substantial returns, are often characterized by high volatility and increased risk. Investors are drawn to these stocks for the possibility of significant gains. Still, they must also be prepared for the market’s fluctuations, which can result in rapid price changes and potential losses.
- Challenges in Identifying Sustainable Growth Companies
Accurately identifying companies capable of sustaining long-term growth is a significant challenge, especially in less developed markets such as Africa. The unpredictability of these markets and limited access to reliable financial data make it difficult for investors to select companies that maintain consistent growth over time.
Basics of Artificial Intelligence and Machine Learning
Overview of AI and ML
Artificial Intelligence (AI) and Machine Learning (ML) are revolutionizing how we live, work, and interact with technology. Here’s an overview:
Artificial Intelligence (AI): Refers to the development of computer systems that can perform tasks that typically require human intelligence, such as:
- Learning
- Problem-solving
- Reasoning
- Perception
- AI systems can analyze vast amounts of data, recognize patterns, and make decisions autonomously
Machine Learning (ML): This subset of AI enables systems to learn from data and improve their performance over time.
ML algorithms can:
- Identify patterns and relationships in data
- Make predictions and forecasts
- Classify and categorize data
- Improve accuracy and efficiency through continuous learning
Together, AI and ML are driving innovation in areas like:
- Natural Language Processing (NLP)
- Computer Vision
- Robotics
- Healthcare
- Finance
As AI and ML continue to evolve, we can expect significant advancements in automation, productivity, and decision-making capabilities, transforming industries and revolutionizing how we live and work.
Definition and Key Concepts
Artificial Intelligence (AI) simulates human intelligence in machines, enabling them to perform tasks that typically require human cognition, such as learning, problem-solving, and decision-making. Machine Learning (ML) is a subset of AI that focuses on developing algorithms that can learn from data, identify patterns, and make predictions or decisions without being explicitly programmed. Key concepts include neural networks, deep learning, natural language processing, and computer vision. ML algorithms can be supervised, unsupervised, or reinforcement-based and are used in applications like image recognition, speech recognition, and predictive analytics, driving innovation and progress in various industries and fields.
Types of AI and ML (Supervised, Unsupervised, Reinforcement Learning)
Artificial Intelligence (AI) and Machine Learning (ML) encompass various data processing and model training approaches. The three primary ML types are supervised learning, unsupervised learning, and reinforcement learning.
Supervised learning is a method where models are trained on a labelled dataset, meaning the data comes with correct output labels. The model learns by comparing its predictions against these labels and adjusting accordingly. This approach is widely used for tasks like classification and regression.
Unsupervised learning, on the other hand, deals with unlabeled data. The model identifies patterns, relationships, or clusters within the data without explicit instructions. It’s commonly applied in clustering, anomaly detection, and dimensionality reduction.
Reinforcement learning involves training a model to make decisions by rewarding desirable outcomes and penalizing undesirable ones. The model learns through trial and error, gradually improving its decision-making to maximize cumulative rewards. This approach is convenient in complex environments like robotics, gaming, and autonomous systems.
How AI and ML are Used in Finance
- Predictive Analytics, Sentiment Analysis, and Algorithmic Trading
Artificial Intelligence (AI) and Machine Learning (ML) are transforming the financial industry through predictive analytics, sentiment analysis, and algorithmic trading applications.
Predictive analytics uses historical data and statistical models to forecast stock prices and market trends, enabling more informed investment decisions.
- Sentiment Analysis
Sentiment analysis leverages natural language processing (NLP) to assess market sentiment by analyzing news articles, social media, and financial reports, helping investors gauge public mood and its potential impact on stock prices.
- Algorithmic Trading
Algorithmic trading automates the trading process, using AI-driven algorithms to execute trades at optimal times based on real-time data and pre-defined strategies.
- Use Cases in Investment Research and Portfolio Management
Artificial Intelligence (AI) and Machine Learning (ML) are crucial in investment research and portfolio management. In investment research, these technologies analyze vast amounts of data to identify lucrative investment opportunities, uncovering trends and patterns that human analysts might miss. In portfolio management, AI and ML optimize portfolios by assessing risk factors, predicting asset performance, and suggesting adjustments to maximize returns while minimizing risks. This leads to more informed, data-driven investment strategies.
Data Sources and Inputs for AI/ML Models
To build accurate AI/ML models for growth stock analysis, it’s essential to feed them with high-quality, relevant data. The following data sources and inputs can be used to train and validate AI/ML models:
Financial Data
- Historical stock prices and trading volumes
- Financial statements (income statements, balance sheets, cash flow statements)
- Earnings reports and analyst estimates
- Dividend yields and payout ratios
Alternative Data
- Social media sentiment and trends
- Web traffic and online engagement metrics
- Industry reports and research studies
- Patents and intellectual property data
- Economic indicators (GDP, inflation, interest rates)
Market Data
- Real-time market prices and quotes
- Trading volumes and order book data
- Market sentiment and volatility metrics
- News and event data (earnings announcements, mergers and acquisitions)
Other Data Sources
- Company filings and regulatory documents
- Management team and board of directors data
- Supply chain and customer data
- Geopolitical and macroeconomic data
When selecting data sources and inputs, consider the following factors:
- Relevance: Is the data relevant to the growth stock being analyzed?
- Accuracy: Is the data accurate and reliable?
- Timeliness: Is the data up-to-date and reflective of current market conditions?
- Completeness: Does the data provide a comprehensive view of the growth stock?
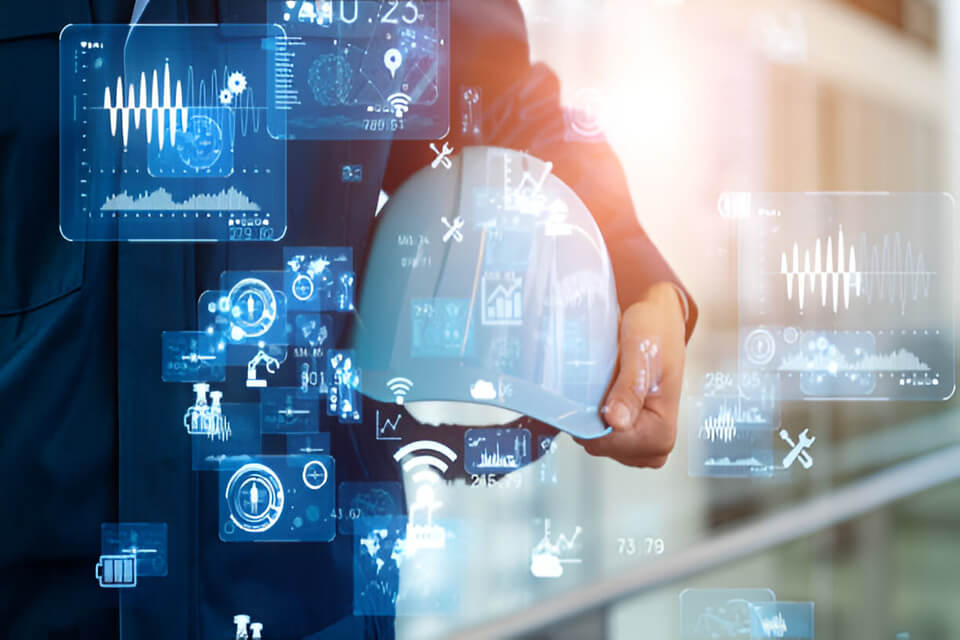
Key Data Types for Growth Stock Analysis
- Financial Statements (Income, Balance Sheet, Cash Flow)
Financial statements are crucial for evaluating a company’s financial health. The income statement details revenues and expenses, showing profitability. The balance sheet provides a snapshot of assets, liabilities, and equity, revealing the company’s financial position. The cash flow statement tracks cash inflows and outflows, indicating liquidity and operational efficiency.
- Historical Stock Price Data and Technical Indicators
Historical stock price data is essential for training AI and ML models to forecast future performance. These models can identify predictive signals by analyzing past price movements, patterns, and trends. Additionally, technical indicators derived from historical data, such as moving averages and RSI, enhance the accuracy of predictions and trading strategies.
- News Sentiment, Earnings Reports, and Analyst Ratings
News sentiment, earnings reports, and analyst ratings are key inputs for AI and ML models assessing market sentiment. Analyzing news sentiment helps gauge investor emotions and market reactions. Earnings reports provide insights into a company’s financial performance, while analyst ratings offer expert evaluations, contributing to more accurate market predictions.
Alternative Data Sources
- Social Media Sentiment, Web Traffic, and Search Trends
Social media sentiment, web traffic, and search trends are valuable non-traditional data sources that provide deeper insights into market dynamics. By analyzing these metrics, businesses can gauge public opinion, track user engagement, and identify emerging interests or concerns, offering a comprehensive view of market trends beyond conventional methods.
- Industry Reports, Patents, and Innovation Metrics
Industry reports, patents, and innovation metrics are crucial for identifying companies leading in technological advancement. Industry reports offer comprehensive market analysis, patents reveal cutting-edge developments, and innovation metrics assess a company’s research and development impact. These tools highlight businesses at the forefront of progress and technological innovation.
- Economic Indicators and Macroeconomic Data
Economic indicators and macroeconomic data offer an essential context for understanding stock performance within the broader economy. Investors gain insights into economic conditions that impact market trends by analyzing factors such as GDP growth, unemployment rates, and inflation. This contextual understanding helps in making more informed investment decisions.
Data Preprocessing and Feature Engineering
- Cleaning, Normalizing, and Transforming Data for Model Input
Cleaning, normalizing, and transforming data are critical steps for preparing data as input for model training. This process ensures data accuracy by removing inconsistencies, scaling values for uniformity, and converting data into a suitable format. Properly processed data enhances model performance and reliability, leading to more accurate predictions and insights.
- Selecting Relevant Features and Reducing Dimensionality
Selecting relevant features and reducing dimensionality are critical aspects of feature engineering, which enhances model performance. You streamline the model’s complexity by identifying and including the most pertinent data points and eliminating less significant ones. This process improves accuracy and efficiency, leading to more effective and interpretable machine learning models.
AI/ML Techniques for Analyzing Growth Stocks
Various AI/ML techniques can be employed to analyze and predict the performance of growth stocks. These techniques are:
Supervised Learning for Predicting Stock Performance
- Regression Models (Linear, Logistic) for Growth Prediction
These models can predict future stock performance by analyzing historical data. They help forecast growth trends and make informed investment decisions based on past patterns.
- Classification Models for Identifying Potential Outperformers
These models are classified by categorizing stocks based on their likelihood of surpassing market performance. They use historical data and patterns to predict which stocks are likely to excel.
- Training Models on Historical Data and Validating Predictions
Models are trained on historical data to learn patterns and trends and then validated by testing their predictions against unseen data. This process ensures the accuracy and reliability of the model’s forecast.
Unsupervised Learning for Market Segmentation
- Clustering Algorithms to Group Similar Growth Stocks
Clustering algorithms use artificial intelligence and machine learning to analyze growth stock characteristics by grouping stocks with similar attributes. This technique helps identify patterns and relationships among stocks, aiding investors in making informed decisions based on grouped similarities.
- Identifying Hidden Patterns and Relationships in Data
Unsupervised learning can identify hidden patterns and relationships in data that are not immediately apparent. This approach reveals underlying structures and trends, providing valuable insights for making informed decisions and predictions.
Sentiment Analysis for Market Sentiment and Stock Trends
- Natural Language Processing (NLP) to Analyze News and Social Media
Natural Language Processing (NLP) techniques analyze the sentiment of news articles and social media posts. This approach helps gauge public opinion and trends, providing valuable insights into market sentiment and potential impacts on stock performance.
- Sentiment Scoring and Its Impact on Stock Prices
Sentiment scoring, using artificial intelligence and machine learning to analyze growth stocks, can significantly impact stock prices. These scores predict market movements by evaluating public sentiment and provide insights into potential price changes and investment opportunities.
Reinforcement Learning for Dynamic Portfolio Management
- Applying Reinforcement Learning to Optimize Growth Stock Portfolios
Applying reinforcement learning to optimize growth stock portfolios involves using algorithms that continually learn and adapt to market fluctuations. These models dynamically adjust portfolio allocations based on real-time data and performance feedback, ensuring investment strategies evolve in response to changing market conditions, enhancing potential returns and minimizing risks.
- Adapting Strategies Based on Market Conditions and Feedback
Adapting strategies based on market conditions and feedback involves leveraging models, continuously learning, and enhancing their performance through new data. These models adjust their approaches dynamically, reflecting real-time insights and trends. This process ensures that strategies remain relevant, effective, and aligned with evolving market demands and consumer preferences.
Building and Training AI/ML Models for Growth Stock Analysis
Building and training AI/ML models involves several steps:
1. Data Preprocessing: Clean, transform, and normalize data to prepare it for modelling.
2. Feature Engineering: Select and create relevant features that capture the underlying patterns in the data.
3. Model Selection: Choose the most suitable AI/ML algorithm for the problem.
4. Model Training: Train the model using the prepared data and hyperparameter tuning.
5. Model Evaluation: Evaluate the model’s performance using accuracy, precision, and recall metrics.
6. Model Deployment: Deploy the trained model in a production-ready environment.
- Tips for Avoiding Common Pitfalls:
1. Data Quality: Ensure high-quality data by handling missing values, outliers, and data drift.
2. Overfitting: Monitor model performance on validation sets to avoid overfitting.
3. Underfitting: Increase model complexity or add more features to avoid underfitting.
4. Hyperparameter Tuning: Use techniques like grid search, random search, or Bayesian optimization to find optimal hyperparameters.
5. Model Interpretability: Understand model decisions using techniques like feature importance, partial dependence plots, or SHAP values.
6. Model Updating: Regularly update models to adapt to changing market conditions and data distributions.
Model Selection and Architecture
- Choosing Appropriate Models Based on Investment Goals
Choosing appropriate models based on investment goals involves selecting models that align with the specific objectives of the investment strategy. This ensures the models effectively support achieving desired financial outcomes and managing risks.
- Considerations for Model Complexity, Accuracy, and Interpretability
Balancing model complexity, accuracy, and interpretability is crucial for creating effective models. While complex models may achieve higher accuracy, ensuring they remain interpretable helps stakeholders understand and trust the results.
Training and Testing the Model
- Splitting Data into Training and Testing Sets
Splitting data into training and testing sets involves dividing the dataset into two parts. The training set builds and trains the model, while the testing set evaluates its performance and generalization.
- Hyperparameter Tuning and Model Optimization
Hyperparameter tuning and model optimization involve adjusting parameters to enhance accuracy and performance. This process refines the model by finding the best settings, leading to improved prediction quality and efficiency.
- Backtesting on Historical Data to Assess Performance
Backtesting involves evaluating models using historical data to assess their performance and reliability. This process helps verify that the models can accurately predict outcomes and perform well under past market conditions.
Model Evaluation and Performance Metrics
- Key Metrics for Evaluating Model Accuracy (e.g., RMSE, Accuracy, Precision)
These metrics are essential for assessing performance because they evaluate how accurately the model predicts outcomes and its reliability across different scenarios, ensuring that predictions are precise and effective for decision-making.
- Analyzing Model Predictions and Identifying Potential Biases
Analyzing model predictions is crucial for identifying and correcting potential biases. Ensuring that models provide fair and accurate results involves scrutinizing data and adjusting algorithms to minimize inaccuracies and enhance reliability.
- Challenges in Collecting and Integrating Diverse Data Sources
Gathering and integrating data from various sources, such as financial statements, market trends, and social media sentiment, can be complex. Data availability and transparency may be limited in many African markets, making it harder to build accurate models.
Applications and Use Cases
Artificial Intelligence (AI) and Machine Learning (ML) have revolutionized stock market analysis, enabling more precise and data-driven investment strategies. These technologies provide powerful tools for filtering, forecasting, and managing portfolios, allowing investors to make informed decisions with enhanced accuracy, speed, and adaptability in dynamic market conditions.
AI-Powered Stock Screeners
AI-powered stock screeners can efficiently analyze vast datasets, identifying and ranking growth stocks based on specific criteria. These tools utilize ML algorithms to evaluate financial metrics, trends, and patterns, helping investors pinpoint high-potential stocks more accurately than traditional methods.
- How AI/ML Models Can Filter and Rank Growth Stocks
AI/ML models filter and rank growth stocks by analyzing factors like earnings growth, valuation ratios, and market trends. These models learn from historical data to identify patterns, enabling investors to discover under-the-radar opportunities and make data-driven investment decisions more efficiently.
- Examples of AI-Driven Stock Screening Tools and Platforms
Popular AI-driven stock screening tools include platforms like Zacks Stock Screener, AlphaSense, and Tickeron. These platforms leverage AI/ML algorithms to provide real-time insights, rankings, and recommendations, enabling investors to identify promising growth stocks and streamline their investment research processes.
Predictive Analytics for Stock Price Forecasting
Predictive analytics utilizes AI/ML to forecast stock prices by analyzing historical data, market trends, and other factors. These models can predict future price movements with remarkable accuracy, giving investors valuable insights into a stock’s growth potential and helping them make informed trading decisions.
- Using AI/ML to Forecast Future Stock Prices and Growth Potential
AI/ML models forecast future stock prices by analyzing historical data, market sentiment, and economic indicators. These models identify patterns and trends, enabling investors to anticipate price movements and growth potential, ultimately leading to more informed and profitable investment decisions.
Sentiment Analysis for Investment Decisions
Powered by AI, Sentiment analysis analyzes market sentiment by processing news, social media, and other sources. It provides a comprehensive view of market trends when integrated with traditional financial metrics, allowing investors to make more informed decisions based on quantitative data and market sentiment.
- Integrating Sentiment Analysis with Traditional Financial Metrics
Integrating sentiment analysis with financial metrics allows investors to gauge market mood and its impact on stock performance. AI tools analyze social media, news, and reports to identify sentiment trends, combining them with financial data to offer a holistic view for making strategic investment decisions.
- Impact of Market Sentiment on Growth Stock Performance
Market sentiment significantly impacts growth stock performance, driving investor behaviour and price fluctuations. AI-driven sentiment analysis helps investors understand these emotional drivers, enabling them to anticipate market movements and adjust their growth stock strategies accordingly.
Dynamic Portfolio Management
Dynamic portfolio management uses AI to optimize and rebalance portfolios in response to market conditions. AI-driven strategies allow investors to adapt to changing trends, minimize risks, and maximize returns by continuously analyzing and adjusting portfolio allocations based on real-time data.
- Using AI to Rebalance and Optimize Growth Stock Portfolios
AI-driven portfolio management tools optimize growth stock portfolios by continuously analyzing market trends, stock performance, and risk factors. These tools automatically rebalance portfolios, ensuring alignment with investment goals and market conditions, thus enhancing potential returns while minimizing risks.
- Strategies for Adapting to Changing Market Conditions
Adapting to changing market conditions involves using AI to monitor real-time data, identify emerging trends, and adjust investment strategies accordingly. AI-driven tools enable investors to react swiftly to market shifts, ensuring their portfolios remain resilient and poised for growth despite volatility.
Challenges and Considerations
Implementing AI/ML in stock market analysis presents challenges like data quality, model overfitting, and ethical concerns. These factors can impact model accuracy and reliability. Additionally, navigating regulatory and compliance issues is essential to ensure AI-driven investment strategies align with industry standards and legal frameworks.
Data Quality and Availability
High-quality, relevant data is crucial for AI/ML model accuracy in stock market analysis. Poor data can lead to inaccurate predictions, affecting investment decisions. Challenges include sourcing, cleaning, and processing alternative data, which may be unstructured or incomplete, complicating its integration into AI-driven models.
- Importance of High-Quality, Relevant Data for Model Accuracy
AI/ML models rely heavily on high-quality, relevant data for accurate stock predictions. Inaccurate or incomplete data can lead to misleading outcomes, potentially resulting in poor investment decisions. Ensuring data integrity is paramount for maximizing the effectiveness of AI-driven financial analysis.
- Challenges in Sourcing and Processing Alternative Data
Sourcing and processing alternative data, such as social media sentiment or satellite imagery, pose significant challenges. This data is often unstructured, requiring sophisticated techniques for cleaning and integration. Moreover, accessing proprietary or real-time data can be expensive, limiting the effectiveness of AI-driven models.
Model Overfitting and Generalization
Overfitting occurs when AI/ML models are too closely tailored to historical data, leading to poor generalization to new data. This can result in inaccurate predictions in real-world scenarios. Ensuring robust and adaptable models is essential for maintaining reliability in dynamic market conditions.
- Risks of Overfitting Models to Historical Data
Overfitting is a critical risk in AI/ML models, where excessive focus on historical data leads to poor performance in real-time markets. This occurs when models become too complex, capturing noise rather than underlying trends, which can result in inaccurate stock predictions and misguided investment decisions.
- Strategies for Ensuring Model Robustness and Adaptability
AI/ML models must be designed for robustness and adaptability to prevent overfitting. Techniques include cross-validation, regularization, and using diverse datasets to ensure models generalize well across different scenarios. Monitoring and updating models are also essential to maintain their effectiveness in evolving markets.
Ethical Considerations and Bias in AI/ML Models
AI/ML models can inadvertently perpetuate biases, leading to unfair or skewed investment decisions. Addressing these biases is crucial to ensure ethical AI use. Additionally, transparency and explainability in AI-driven decisions are vital to maintaining trust and accountability in financial markets.
- Addressing Potential Biases in AI/ML Models
Bias in AI/ML models can arise from unrepresentative training data or flawed algorithms, leading to unfair or skewed outcomes. To address this, developers must ensure diverse, balanced datasets and implement bias detection techniques, fostering fairness and accuracy in AI-driven financial analysis.
- Ensuring Transparency and Explainability in Investment Decisions
Transparency and explainability in AI/ML models are essential for ethical investment decisions. Investors and regulators must understand how models make predictions to ensure trust and accountability. Implementing explainable AI (XAI) techniques helps demystify complex models, making decision-making more transparent.
Regulatory and Compliance Issues
Stringent regulations govern the use of AI/ML in finance to ensure fair, transparent, and compliant practices. Financial institutions must navigate these regulatory landscapes to avoid penalties and ensure their AI-driven strategies align with industry standards and legal requirements.
- Overview of Regulations Governing AI/ML Use in Finance
Regulations governing AI/ML in finance include data privacy laws, algorithmic transparency requirements, and guidelines for ethical AI use. These rules ensure that AI-driven models adhere to legal standards, preventing misuse or discriminatory practices in financial markets and safeguarding investor interests.
- Ensuring Compliance with Industry Standards and Guidelines
Ensuring compliance with industry standards and regulatory guidelines is crucial for AI/ML applications in finance. Financial institutions must continuously monitor evolving regulations, implement robust governance frameworks, and regularly audit their AI models to avoid legal repercussions and maintain market integrity.
Future Trends in AI/ML and Growth Stock Analysis
The future of growth stock analysis will see AI/ML technologies becoming more advanced and integrated with other cutting-edge tools. As these technologies evolve, the role of human expertise will shift, balancing AI-driven insights with experienced judgment to create more robust and dynamic investment strategies.
Advancements in AI/ML Technologies
Emerging AI/ML trends in financial analysis include improved natural language processing, automated decision-making, and enhanced predictive models. These advancements will lead to more accurate growth stock predictions. Additionally, the potential integration of quantum computing could revolutionize how AI processes complex financial data, making analysis faster and more precise.
- Emerging Trends in AI/ML for Financial Analysis
Emerging trends in AI/ML for financial analysis include deep learning for more accurate stock predictions, AI-driven sentiment analysis from social media, and automated trading systems. These innovations promise to enhance growth stock analysis’s precision, speed, and efficiency, leading to better investment outcomes.
- Potential Impact of Quantum Computing on Growth Stock Analysis
Quantum computing could drastically alter growth stock analysis by enabling AI models to simultaneously process vast amounts of data, solving complex problems in seconds. This would significantly enhance predictive accuracy and optimize investment strategies, providing investors with unprecedented insights and a competitive edge in dynamic markets.
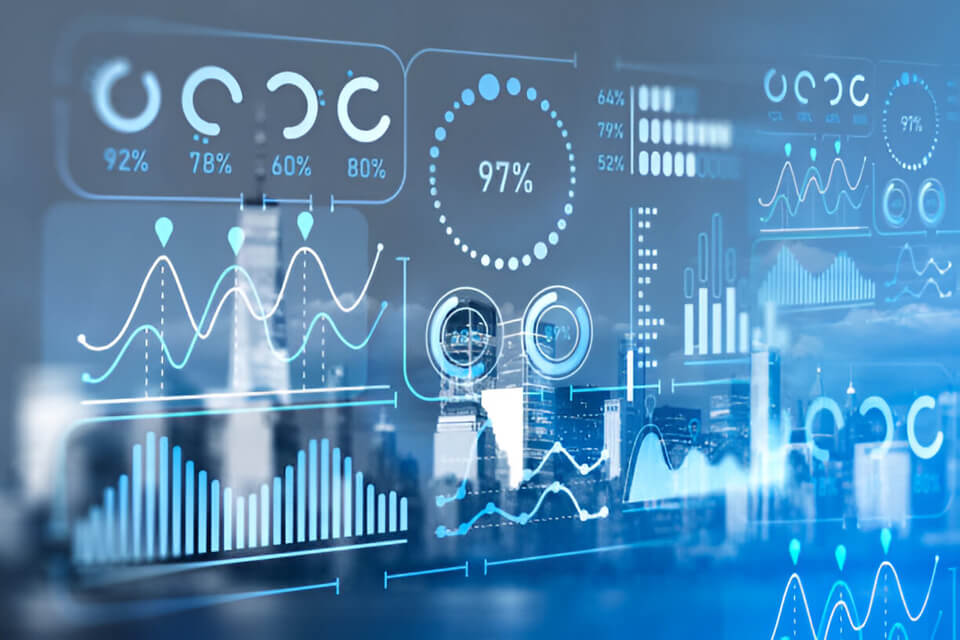
Integration of AI/ML with Other Technologies
Integrating AI/ML with blockchain, IoT, and big data analytics can create more sophisticated investment platforms. These technologies offer enhanced data security, real-time insights, and comprehensive market analyses, paving the way for AI-driven platforms offering more accurate and holistic growth stock analysis.
- Combining AI/ML with Blockchain, IoT, and Big Data Analytics
The fusion of AI/ML with blockchain, IoT, and big data analytics offers a powerful synergy in growth stock analysis. Blockchain ensures data integrity, IoT provides real-time data, and big data analytics enables in-depth market analysis. Together, they form a comprehensive AI-driven investment platform with enhanced security and accuracy.
- Prospects for AI-Driven Investment Platforms
AI-driven investment platforms are poised for significant growth, offering personalized investment strategies, real-time analysis, and automated trading. As AI integrates with technologies like blockchain and big data, these platforms will become more robust, providing investors with advanced tools to navigate complex markets and identify high-potential growth stocks.
The Role of Human Expertise in AI-Driven Investing
Human expertise remains vital in AI-driven investing, offering nuanced insights, strategic judgment, and ethical considerations that machines can’t fully replicate. As AI/ML models become more sophisticated, financial analysts will increasingly focus on interpreting AI outputs and making decisions that balance data-driven analysis with human experience.
- Balancing AI/ML Models with Human Judgment and Experience
Balancing AI/ML models with human judgment is essential for effective investing. While AI excels in data analysis and pattern recognition, human expertise is crucial for interpreting nuanced market conditions, ethical considerations, and long-term strategies. Together, they create a more holistic and adaptive investment approach.
- The Evolving Role of Financial Analysts in an AI-Powered World
In an AI-powered world, financial analysts will evolve from data crunchers to strategic advisors, focusing on interpreting AI-driven insights and making complex decisions. Their expertise in understanding market dynamics and AI’s analytical power will be vital in shaping successful, balanced investment strategies.
Case Study and Real-World Examples
Successful AI/ML Applications in Growth Stock Investing
Hedge Fund Example
Renaissance Technologies, a leading hedge fund, uses AI/ML to analyze vast data and identify profitable trades. Their Medallion Fund has consistently outperformed the market, with returns of over 30% per year.
Robo-Advisor Example
Wealthfront, a robo-advisor, uses AI/ML to optimize investment portfolios and provide personalized financial planning. They have over $20 billion in assets under management and have consistently outperformed traditional financial advisors.
Platform Example
QuantConnect, an open-source platform, allows users to build and deploy AI/ML-based trading strategies. Their users have developed strategies that have consistently outperformed the market.
Analysis of AI-driven Growth Stock Portfolios and Their Performance
- A study by McKinsey found that AI-driven portfolios outperformed traditional portfolios by 2-3% per year.
- A study by Harvard Business Review found that AI-driven portfolios were more diversified and had lower risk than traditional portfolios.
Lessons Learned from AI/ML Failures in Finance
- Knight Capital Group: In 2012, Knight Capital Group’s AI/ML-based trading system failed, causing a $460 million loss. The failure was due to a lack of testing and validation of the system.
- Long-Term Capital Management: In 1998, Long-Term Capital Management’s AI/ML-based trading system failed, causing a $4.6 billion loss. The failure was due to over-reliance on complex models and a lack of risk management.
Frequently Asked Questions
1. What is the role of AI in stock market analysis?
AI enhances stock market analysis by processing vast amounts of financial data, identifying patterns, predicting stock performance, and providing insights into market trends. It helps investors make data-driven decisions by analyzing historical data, sentiment, and other relevant factors.
2. How can Machine Learning improve growth stock predictions?
Machine Learning improves growth stock predictions using algorithms to analyze historical stock data, financial statements, and alternative data sources. It can identify patterns and trends that may not be apparent through traditional methods, leading to more accurate predictions of stock performance.
3. What types of data are AI models used to analyze growth stocks?
AI models use various data types, including financial statements (income, balance sheet, cash flow), historical stock price data, technical indicators, news sentiment, earnings reports, and analyst ratings. Additionally, alternative data sources like social media sentiment and web traffic can provide further insights.
4. How does sentiment analysis contribute to stock market predictions?
Sentiment analysis evaluates market sentiment by analyzing news articles, social media, and financial reports. It helps gauge public opinion and investor sentiment, impacting stock prices and trends. By understanding sentiment, AI models can predict market movements more accurately.
5. What are the limitations of using AI and ML for stock analysis?
Limitations include the risk of overfitting, where models may perform well on historical data but poorly on new data. Additionally, AI models can be complex and hard to interpret, potentially leading to a lack of transparency. Data quality and availability can also impact the effectiveness of AI and ML models.
Conclusion
AI and ML have transformed the landscape of growth stock analysis, offering powerful tools for identifying investment opportunities and optimizing portfolios. For investors in Africa and other emerging markets, these technologies provide a means to overcome challenges such as data scarcity and market volatility. However, successfully implementing AI and ML in finance requires careful consideration of data quality, model interpretability, and ethical implications.
As AI and ML evolve, they will undoubtedly play an increasingly significant role in the financial markets, offering new opportunities for investors to achieve their goals. Investors can leverage these technologies to gain a competitive edge in pursuing growth stock potential by staying informed about the latest advancements and trends.